Decoding Profound Fortification Learning: Q-Learning and the AI Revolution
Artificial intelligence (AI) has permeated numerous facets of our lives, and reinforcement learning (RL) stands as a pivotal force behind its evolution. Reinforcement learning, in essence, empowers AI agents to learn by interacting with their environment, receiving feedback in the form of rewards or penalties, and subsequently refining their actions to maximize cumulative rewards. In this blog post, we will delve into the realm of deep reinforcement learning, exploring the concept of Q-learning and its profound implications for the AI landscape.
Let’s connect
Book a meeting
Understanding Reinforcement Learning
At its core, reinforcement learning draws inspiration from how humans and animals learn through trial and error. Imagine a child learning to ride a bicycle; they experiment with different actions, receiving positive reinforcement (praise or a sense of accomplishment) for successful attempts and negative reinforcement (falls or struggles) for unsuccessful ones. Over time, the child refines their motor skills and learns to maintain balance, propelled by the desire to maximize the positive reinforcement.
Similarly, in AI reinforcement learning, an agent operates within an environment, taking actions and receiving rewards or penalties based on the outcomes. The agent’s objective is to find a policy—a technique for selecting actions—that optimizes its total remunerate over time.
The Power of Deep Reinforcement Learning
Profound support learning (DRL) speaks to an intriguing crossing point of fortification learning and profound learning. In DRL, deep neural networks are employed to approximate the value function—a measure of the expected cumulative reward an agent can achieve from a given state—or the policy directly.
One of the most celebrated DRL algorithms is Q-learning. In Q-learning, the operator keeps up a Q-table, which stores Q-values for each state-action combined. These Q-values appraise the anticipated aggregate compensation for taking a specific activity in a particular state. The agent iteratively updates these Q-values based on its experiences, gradually converging towards an optimal policy.
Q-Learning in Action: AI Reinforcement Learning Applications
Q-learning and deep reinforcement learning have found applications in a diverse range of fields, showcasing their transformative potential:
- Robotics: DRL has empowered robots to master intricate tasks, from manipulating objects with dexterity to navigating complex environments autonomously. By interacting with the physical world and receiving feedback, robots can learn to optimize their actions for specific goals, such as assembling products or performing surgical procedures.
- Gaming: DRL has accomplished surprising breakthroughs in the gaming space. AI agents powered by DRL algorithms have surpassed human performance in games like Go, chess, and even complex video games like Dota 2. This underscores the potential of DRL to handle perplexing decision-making challenges.
- Autonomous Vehicles: The development of self-driving cars heavily relies on reinforcement learning. DRL algorithms enable vehicles to learn the nuances of driving, from obeying traffic rules to making split-second decisions in dynamic environments. By interacting with the road and receiving feedback from sensors, autonomous vehicles can continuously improve their driving capabilities.
These advantages are driving the rapid adoption of AI robotics across a wide spectrum of industries. From car manufacturing to delicate medical procedures, AI robots are transforming the way we work and creating a future where humans and intelligent machines collaborate seamlessly.
Challenges and Future Directions
While AI reinforcement learning and Q-learning have demonstrated remarkable progress, they still face certain challenges. The sample efficiency of DRL algorithms remains a concern, as they often require a substantial amount of interaction with the environment to learn effectively. Moreover, guaranteeing the security and vigor of DRL specialists in real-world scenarios posture continuous inquire about challenges.
Looking ahead, the future of reinforcement learning appears incredibly promising. As research progresses, we can anticipate DRL algorithms becoming more sample efficient, capable of learning from fewer interactions. Furthermore, the integration of DRL with other AI techniques, such as natural language processing and computer vision, holds the potential to create even more sophisticated and versatile AI agents.
Conclusion
Reinforcement learning, deep reinforcement learning, Q-learning, and AI reinforcement learning collectively represent a paradigm shift in how we approach artificial intelligence. By mimicking the trial-and-error learning process inherent in nature, RL empowers AI agents to learn, adapt, and excel in complex environments. The applications of DRL span a vast spectrum, from revolutionizing robotics and gaming to advancing the development of autonomous vehicles. As research continues to unravel the intricacies of reinforcement learning, we can envision a future where AI agents exhibit unprecedented levels of intelligence, autonomy, and problem-solving capabilities.
Global success stories
Here are some related content that highlight our capability in delivering AI solutions that save costs as well as boost productivity.
Manufacturing 4.0:
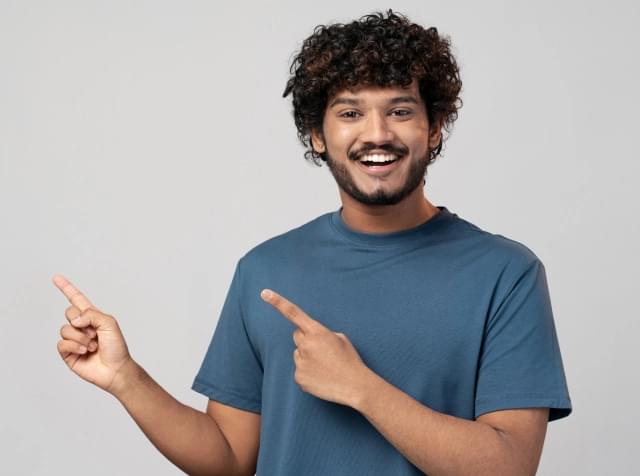